

While the SML has been assessed on local and regional scales 12, 15, global-scale datasets remain sparse.

Recently, machine learning (e.g., K-nearest neighbor, random forest and neural networks) has been applied to resolve important questions in oceanography, including the global distribution of seafloor total organic carbon, benthic properties, sediment porosity/density and sediment accumulation rates 25, 26, 27, 28, 29, 30. Some examples of neural networks applications in the earth sciences include: hydrology, including flooding forecasts, and water quality modeling 19, 20 geophysics/geomorphology, including earthquake predictions, and simulating land-use change 21, 22 and atmospheric sciences, by modeling cloud formation and temperatures 23, 24. Neural networks have emerged as a powerful tool to resolve complex spatial and temporal patterns that are common in large datasets in the geosciences 17, 18. Thus, the distribution and thickness of the SML are important to improve global flux estimates of dissolved and particulate constituents of key biogeochemical cycles in Earth System Models 16. Particle reworking and remobilization can result in loss of chronological information 13, 14, 15 and is highly heterogeneous spatially and temporally. In rapidly changing coastal environments, sediments are commonly remobilized as stationary fluid muds, and/or resuspended and laterally transported as dense suspensions 11, 12. Pioneering work using 210Pb for dating marine sediments 7, established chronologies in estuarine and coastal sediments 8, 9, 10.
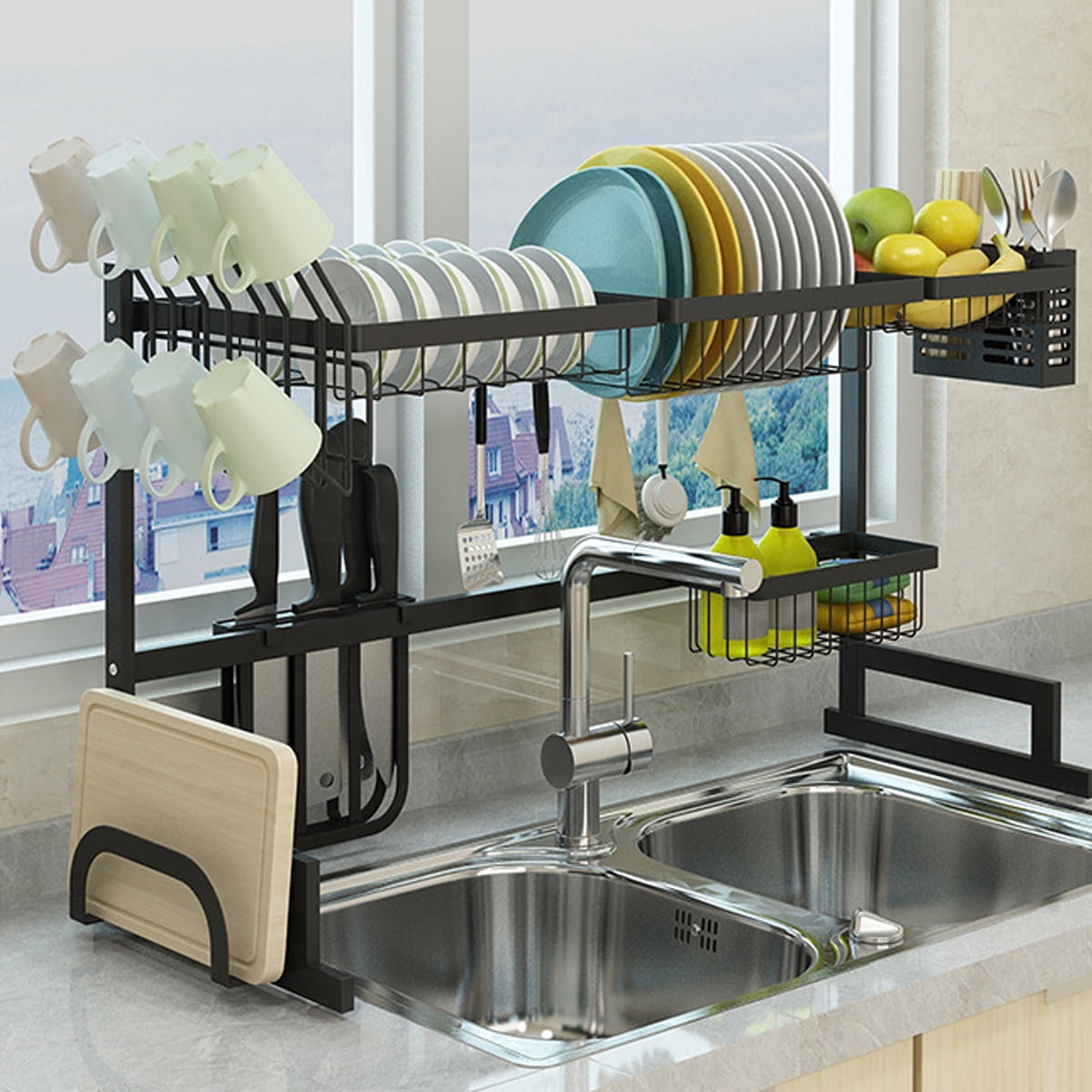
Atmospherically derived 210Pb (half-life of 22.3 years) is a natural “tracer” for sediments accumulating over time. Quantifying the thickness of the SML is challenging, particularly in highly dynamic coastal sediments.
#Over the sink shelf driver#
For instance, the SML is an important driver of the exchange of nutrients, organic carbon, redox-sensitive elements and greenhouse gases between the seafloor and the overlying seawater 5, 6. Constraining the thickness and location of the sediment mixed layer (SML) is essential for resolving key pathways in marine biogeochemical cycles 3, 4. These sediment records can be altered by physical and/or biological mixing which can modify sedimentary structures and obscure record interpretations 2. Our global scale observations reveal that reworking can accelerate organic matter degradation and reduce carbon storage in coastal sediments.Ĭoastal sediments record detailed historical changes of land-use and climate, which can impact source-to-sink particle dynamics across the land-ocean boundary 1. Sites with SML thickness >60 cm usually have lower organic carbon accumulation rates (<50 g C m −2 yr −1) and total organic carbon/specific surface area ratios (<0.4 mg m −2). Organic carbon preservation and SMLs are inversely related as mixing stimulates oxidation in sediments which enhances organic matter decomposition. We show that highly dynamic regions such as large estuaries have thicker SMLs than most oceanic sediments. Here we map the global distribution of the coastal SML based on excess 210Pb ( 210Pb ex) profiles and then use a neural network model to upscale these observations.

Processes in the sediment mixed layer (SML) control the transfer and reactivity of both particulate and dissolved matter in coastal interfaces. The sediment-water interface in the coastal ocean is a highly dynamic zone controlling biogeochemical fluxes of greenhouse gases, nutrients, and metals.
